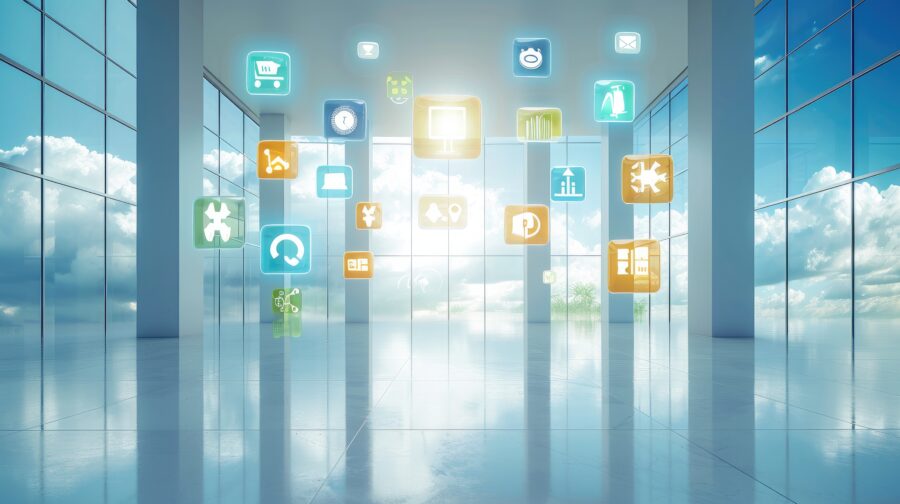
Overview: Data value creation in companies
For a long time, everyone was talking about data. “The world’s most valuable resource is no longer oil, but data” (The Economist, 2017) was the ubiquitous mantra. However, advances in the field of artificial intelligence (AI) are currently dominating the media, driven in particular by generative AI such as ChatGPT, DALL-E and the like, which can create new content such as text, images or speech. These generated data instances are in turn created with the help of technology on the basis of enormous data sets (Touati, 2024). Accordingly, it is becoming increasingly relevant for companies today and in the future to deal with the topic of “data” as the basis for value-adding activities. The Competence Center (CC) Ecosystems of the Business Engineering Institute St. Gallen develops solutions for relevant practical problems and addresses, among other things, the utilization of data as a key factor for business success in dynamic environments. This blog post provides an introduction to the research in the field of data value creation for companies at the CC ecosystems.
Today, “most organizations are awash in data” (Wixom and Ross, 2017, p. 10). This also applies to banks, which hold large volumes of data that are particularly worthy of protection, especially their customers’ personal and financial data. As the amount of data grows, the opportunities for companies to leverage this data also multiply (Baecker, Böttcher and Weking, 2021). Nevertheless, there are numerous regulatory requirements and ethical reservations that limit these opportunities, especially for banks. Nevertheless, the utilization of data represents “one of the most significant technological disruptions for companies” (Chen et al., 2017, p. 19). This potential can also be demonstrated empirically, with companies that leverage data outperforming others in terms of productivity and profitability (McAfee and Brynjolfsson, 2012; Quaadgras, Weill and Ross, 2014; Wiener, Saunders and Marabelli, 2020).
As a result, companies are coming under increasing pressure to “extract as much potential as possible from their data” in order to maintain their current market position or even develop a competitive advantage (Chen et al., 2017; Grover et al., 2018). In this context, completely new data-driven business models are often to be developed that build on data as a core resource (Hartmann et al., 2016; Schüritz and Satzger, 2016). According to this dogma, companies may be able to sell their existing data directly to interested parties, which is at least to a certain extent independent of the company’s previous services. For example, it is common practice for retailers to sell data from their customers’ sales transactions to consumer research companies (Wixom, 2014). The provision of data via application programming interfaces (APIs) is also becoming increasingly important and can represent a profitable business model for companies (Mobey Forum 2022). On the other hand, companies can dedicate themselves to the creation and distribution of more complex data or information products (Quach et al., 2022) for example by developing AI models adapted to a specific purpose.
However, as the development of entirely new business models is unlikely to be a viable practice for every company, data does not have to remain unused. Data can also be used for incremental improvements to the existing business in various ways (Chen, Chiang and Storey, 2012). For example, processes can be automated or made more efficient by collecting information about their workflows and interfaces (Möller et al., 2020). On the other hand, detailed information can be used to better assess and reduce a company’s risks (Borthakur, Srinivasan and Kulkarni, 2022). For example, potential debtors are checked in terms of their creditworthiness in order to reduce the default risk of issued loans. Corporate decisions can also be supported by making them less based on gut feelings and more information-based and well-considered (Breidbach and Maglio, 2020) for example, by analyzing the potential market, competitors and other factors when making investment decisions. Such approaches are also used in customer interaction. For example, customer needs and their behavior can be analyzed in order to better understand the customer at the heart of the company in the first instance (Rantala et al., 2020) but also to manage targeted advertising campaigns for specific customer groups or even to develop customized products and services (Fast, Schnurr and Wohlfarth, 2021).
There seem to be almost limitless opportunities for companies to create value from data. However, this bouquet of possibilities often presents companies and decision-makers with problems in selecting the “right one(s)” of these possibilities (Nutt, 2004). In particular, it should be noted that the implementation of data usage scenarios typically involves significant investments for companies (Grover et al., 2018) for example, through the necessary provision of hardware and software to guarantee storage options, access and availability of data, as well as ensuring data quality that is appropriate for the purposes of use. In addition to these technical aspects, the use of data requires further organizational skills in the context of data management, ranging from the preprocessing of data, its analysis and evaluation to the use of the findings or their monetization.
To justify potential investments accordingly, companies first and foremost need clarity about the opportunities available to them to create value from data (Grover et al., 2018). Since the existing scientific literature, which is fragmented across numerous areas such as data monetization, data-driven business models and business analytics, does not provide a comprehensive solution to this problem, a solution artefact is being developed as part of the CC Ecosystem that comprehensively identifies the possible options for creating value from data for companies and classifies them within a systematization framework to make it easier for decision-makers to make a choice. This solution artifact will be presented in further blog posts. More to come …
References
Baecker, J., Böttcher, T.P. and Weking, J. (2021) ‘How Companies Create Value From Data – A Taxonomy on Data, Approaches, and Resulting Business Value’, ECIS 2021 Research Papers [Preprint]. Available at: https://aisel.aisnet.org/ecis2021_rp/124.
Borthakur, M., Srinivasan, H. and Kulkarni, P. (2022) ‘Monetizing Data Points to Increase Profitability for Banks’, Cardiometry, (24), pp. 948-959. Available at: https://doi.org/10.18137/cardiometry.2022.24.948959.
Breidbach, C.F. and Maglio, P. (2020) ‘Accountable algorithms? The ethical implications of data-driven business models’, Journal of Service Management, 31(2), pp. 163-185. Available at: https://doi.org/10.1108/JOSM-03-2019-0073.
Chen, H., Chiang, R.H.L. and Storey, V.C. (2012) ‘Business Intelligence and Analytics: From Big Data to Big Impact’, MIS Quarterly, 36(4), pp. 1165-1188. available at: https://doi.org/10.2307/41703503.
Chen, H.-M. et al. (2017) ‘How Lufthansa Capitalized on Big Data for Business Model Renovation’, MIS Quarterly Executive, 16(1), pp. 19-34.
Fast, V., Schnurr, D. and Wohlfarth, M. (2021) Data-driven Competitive Advantages in Digital Markets: An Overview of Data Value and Facilitating Factors, Wirtschaftsinformatik 2021 Proceedings. Available at: https://aisel.aisnet.org/wi2021/GFuture18/Track18/9.
Grover, V. et al. (2018) ‘Creating Strategic Business Value from Big Data Analytics: A Research Framework’, Journal of Management Information Systems, 35(2), pp. 388-423. Available at: https://doi.org/10.1080/07421222.2018.1451951.
Hartmann, P.M. et al. (2016) ‘Capturing value from big data – a taxonomy of data-driven business models used by start-up firms.’, International Journal of Operations & Production Management, 36(10), pp. 1382-1406. Available at: https://doi.org/10.1108/IJOPM-02-2014-0098.
McAfee, A. and Brynjolfsson, E. (2012) ‘Big Data: The Management Revolution’, Harvard Business Review, 90(10), pp. 61-67.
Mobey Forum (2022) ‘Mobey Forum Report November 2022: Monetization of Open Banking APIs, https://mobeyforum.org/wp-content/uploads/2022/11/Monetisation-of-Open-Banking-APIs-report-by-Mobey-Forums-Open-Banking-Expert-Group.pdf, retrieved on: 08.04.2024.
Möller, F. et al. (2020) Data-driven Business Models in Logistics: A Taxonomy of Optimization and Visibility Services, Hawaii International Conference on System Sciences 2020 (HICSS-53). Available at: https://aisel.aisnet.org/hicss-53/os/org_issues_in_bi/5.
Nutt, P.C. (2004) ‘Expanding the search for alternatives during strategic decision-making’, Academy of Management Perspectives, 18(4), pp. 13-28. Available at: https://doi.org/10.5465/ame.2004.15268668.
Quaadgras, A., Weill, P. and Ross, J.W. (2014) ‘Management commitments that maximize business impact from IT’, Journal of Information Technology, 29(2), pp. 114-127. Available at: https://doi.org/10.1057/jit.2014.7.
Quach, S. et al. (2022) ‘Digital technologies: tensions in privacy and data.’, Journal of the Academy of Marketing Science, 50(6), pp. 1299-1323. Available at: https://doi.org/10.1007/s11747-022-00845-y.
Rantala, T. et al. (2020) ‘Selling Data-Based Value in Business-to-Business Markets’, Technology Innovation Management Review, 10(1), pp. 45-53.
Schüritz, R. and Satzger, G. (2016) ‘Patterns of Data-Infused Business Model Innovation’, in. 2016 IEEE 18th Conference on Business Informatics (CBI), pp. 133-142. Available at: https://doi.org/10.1109/CBI.2016.23.
The Economist (2017). ‘The world’s most valuable resource is no longer oil, but data.’ 07.05.2017. https://www.economist.com/leaders/2017/05/06/the-worlds-most-valuable-resource-is-no-longer-oil-but-data, accessed on: 12.09.2023.
Touati, B. (2024) ‘Generative AI’, Technopedia, 15.02.2024. https://www.techopedia.com/de/definition/generative-ki, accessed 05.04.2024.
Wiener, M., Saunders, C. and Marabelli, M. (2020) ‘Big-data business models: A critical literature review and multiperspective research framework’, Journal of Information Technology, 35(1), pp. 66-91. Available at: https://doi.org/10.1177/0268396219896811.
Wixom, B.H. (2014) ‘Cashing In on Your Data’, Research Briefing. MIT CISR [Preprint]. Available at: https://cisr.mit.edu/publication/2014_0801_DataMonetization_Wixom (Accessed: September 29, 2023).
Wixom, B.H. and Ross, J.W. (2017) ‘How to Monetize Your Data’, MIT Sloan Management Review, 58(3), pp. 10-13.
- Overview: Data value creation in companies - 09.04.2024
- Study results analyzing the needs of Swiss individuals for trusted data spaces - 11.10.2023
- The potential and development of data spaces - 31.07.2023