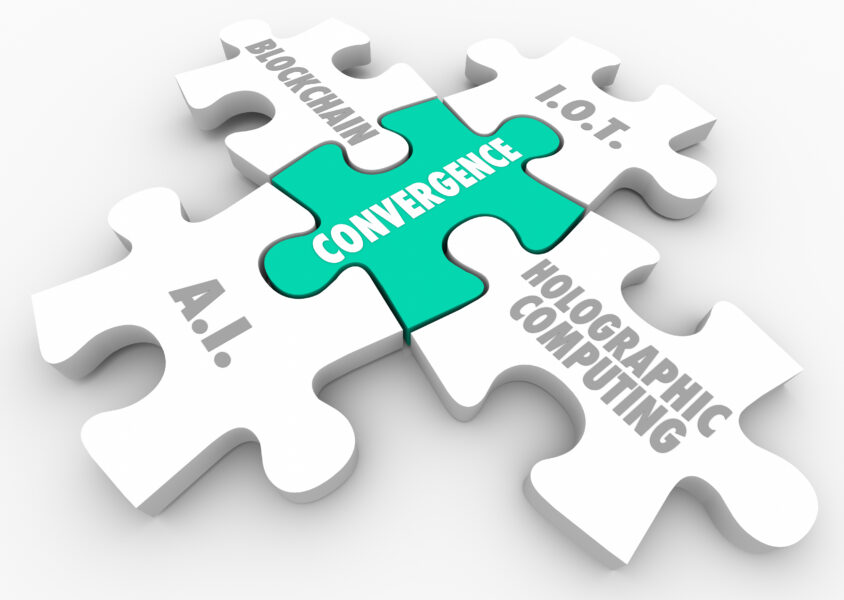
The Convergence of Distributed Ledger Technology and Artificial Intelligence Exemplified by the Lending Process (Part 1)
The distributed ledger technology (DLT) and artificial intelligence (AI) are two promising technologies that are at the top of their hype cycle. Although the idea of AI took shape as early as 1955 at the Dartmouth Conference and the blockchain (BC) and the higher-level DLT concept did not gain wider attention until 2009 due to the Bitcoin protocol (McCarthy et al., 1955; Nakamoto, 2008), both AI and DLT today offer innovative possibilities for process optimization in companies (Zachariadis et al., 2019), especially in bank-related application areas. Scientists assume that the convergence of the blockchain, the Internet of Things (IoT) and artificial intelligence offers considerable opportunities, such as accelerating the pace of service, process and business model innovations (Hughes et al., 2019). However, the information available in the current literature and research on how such an integration could be implemented in practice is still sparse. Over the next week, this series of articles will explore how specific elements of DLT and AI can be combined to achieve potential technological convergence, and what significance this convergence has for the lending process – representative of all those processes in or outside the financial industry that are suitable for simultaneous application of the two technologies. In this first article, we explain why it makes sense to combine artificial intelligence with blockchain technology and what, of all things, makes the lending process an ideal use case for the convergence of the two technologies. In the second article we analyze to what extent the lending process can be optimized by using AI and DLT and in article three we present the process characteristics that indicate that a process could benefit from a joint use of DLT and AI.
State of research on the convergence of DLT and AI
The original concept of convergence, which originated in evolutionary biology, describes the tendency for unrelated units to develop into a single entity with new characteristics under similar environmental conditions (Stayton, 2015). In current practice, the concept refers to the integration of two or more different technologies into a single device or system, which makes it possible to perform multiple and new tasks as they evolve and progress (European Commission, 1997). In the case of DLT and AI, it is crucial to understand that their capabilities do not exist in a vacuum and are intertwined with each other’s technology development. The current literature on the convergence of DLT and AI is still scarce and presents different views on this issue. However, conclusions of current research suggest that AI and DLT differ in several ways:
- AI is traditionally driven by centralized infrastructures, as opposed to the decentralized and distributed nature of DLT (Corea, 2019)
- AI is more of a black box solution (Castelvecchi, 2016) and DLT tends to be more transparent (Beck et al., 2016)
- AI is often based on probabilistic formulas (Hutter, 2004), while DLT is characterized by a more deterministic logic (Smart Contracts) (Cachin, 2016)
It is argued that the two technologies have opposite characteristics: While DLT has security problems and weaknesses in terms of scalability and efficiency, the data used by AI is not always trustworthy, especially in terms of transparency and privacy (Cath, 2018). AI helps people understand and analyze huge amounts of data, but because the results are stored centrally, they can be hacked and abused. DLT, on the other hand, is not suitable for analyzing data, but it is suitable for decentralized storage of data sets and can protect AI input or output data, resulting in increased security and faster and more transparent processes (Rabah, 2018). Therefore, the convergence of both technologies could reshape current processes and enable improved or completely new services (Dinh and Thai, 2018). Both technologies complement each other and have capabilities that overcome the weaknesses of the other technology (Dinh and Thai, 2018). The architecture of DLT includes different implementation options (private vs. public DLTs) and trade-offs between security, performance and decentralization. In theory, AI improves the resolution of these conflicting goals by the targeted analysis of large amounts of data and optimizes the consistency of transactional data, which should be stored unalterably on the DLT. (Pinto, 2018)
The end-to-end lending process
The financial services sector is often regarded as one of the most important industries for early application of DLT and AI. For example, financial institutions were among the first to use prototypes for decentralized peer-to-peer payment traffic similar to the Bitcoin protocol or chatbots for digital customer interaction. Many financial institutions have recognized that mastering technology is crucial to remain competitive in a globalized economy (Lagarde, 2018). Credit products are one of the main pillars of banks’ business model, so the analysis focuses on the end-to-end lending process shown in Figure 1.

In fact, it involves (1) interactions between the client advisor, the client and bank employees, (2) includes analytical tasks as part of the decision-making process and (3) constitutes a case where sensitive information, such as the financial situation of the client, is transferred between different parties. Both the communication between different parties and the need for data analysis make this process a candidate for the use of AI, while the transfer of sensitive information and the execution of transactions are among the capabilities of the blockchain. Therefore, the lending process is a good example of how the two technologies can be combined to optimize a task that is traditionally performed manually, is time and resource intensive, and is highly error-prone due to the transfer of data between different entities.
How exactly AI and DLT can optimize the lending process will be analyzed early next week in the second part of this series.
Sources:
McCarthy, J., Minsky, M. L., Rochester, N. and Shannon, C.E. (1955). “A Proposal for the Darth-mouth Summer Research Project on Artificial Intelligence.” Darthmouth College, New Hampshire.
Nakamoto, S. (2008). Bitcoin: A peer-to-peer electronic cash system.
Zachariadis, M., Hileman, G. and Scott, S. V. (2019). “Governance and control in distributed ledg-ers: Understanding the challenges facing blockchain technology in financial services.” Infor-mation and Organization 29 (2), 105–107.
Hughes et al., (2019). Zhao, J.L., Fan, S. and Yan, J. (2016). “Overview of business innovations and research opportunities in blockchain and introduction to the special issue.” Financial Innovation 2 (28), 1–7.
Lagarde, C. (2018). “Central Banking and Fintech: A Brave New World.” Innovations: Technology, Governance, Globalization 12 (1-2), 4–8.
Alt, R. and Puschmann T. (2016). Digitalisierung der Finanzindustrie. Berlin/Heidelberg: Springer
Stayton C. T. (2015). “What does convergent evolution mean? The interpretation of convergence and its implications in the search for limits to evolution.” Interface focus 5 (6), 20150039.
European Commission (1997), Green Paper on the convergence of the telecommunications, medi-a and information technology sectors, and the implications for regulation. COM (97) 623. Brussels.
Corea, F. (2019). “Applied Artificial Intelligence: Where AI Can Be Used In Business.” Springer In-ternational Publishing 1.
Castelvecchi, D. (2016). “Can we open the black box of AI?.” Nature News 538 (7623), 20–23.
Beck, R., Avital, M., Rossi, M. and Thatcher, J. B. (2017). “Blockchain technology in business and information systems research.” Business & Information Systems Engineering 59 (6), 381–384.
Hutter, M. (2004). Universal artificial intelligence: Sequential decisions based on algorithmic proba-bility. Springer Science & Business Media.
Cachin, C. (2016). Architecture of the hyperledger blockchain fabric. In: Workshop on distributed cryptocurrencies and consensus ledgers 310, p. 4.
Cath, C. (2018). “Governing artificial intelligence: ethical, legal and technical opportunities and challenges.” Philosophical Transactions of the Royal Society A: Mathematical, Physical and Engi-neering Sciences 276 (2133).
Dinh, T. and Thai, M. (2018). “AI and Blockchain: A Disruptive Integration.” Computer 51 (9), 48–53.
Pinto, R. (2018). Next Steps In The Integration Of Artificial Intelligence And The Blockchain. URL: https://www.forbes.com/sites/forbestechcouncil/2018/10/09/next-steps-in-the-integration-of-artificial-intelligence-and-the-blockchain/#141519ea3273/ (visited on 11/20/2019)